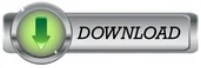
Even so, they are useful in different frameworks: kriging is made for estimation of a single realization of a random field, while regression models are based on multiple observations of a multivariate data set. Both theories derive a best linear unbiased estimator based on assumptions on covariances, make use of Gauss–Markov theorem to prove independence of the estimate and error, and use very similar formulae. The method is closely related to regression analysis. Kriging predicts the value of a function at a given point by computing a weighted average of the known values of the function in the neighborhood of the point. Main principles Related terms and techniques

The method is widely used in the domain of spatial analysis and computer experiments. Interpolating methods based on other criteria such as smoothness (e.g., smoothing spline) may not yield the BLUP. Under suitable assumptions of the prior, kriging gives the best linear unbiased prediction (BLUP) at unsampled locations. In statistics, originally in geostatistics, kriging or Kriging, also known as Gaussian process regression, is a method of interpolation based on Gaussian process governed by prior covariances.
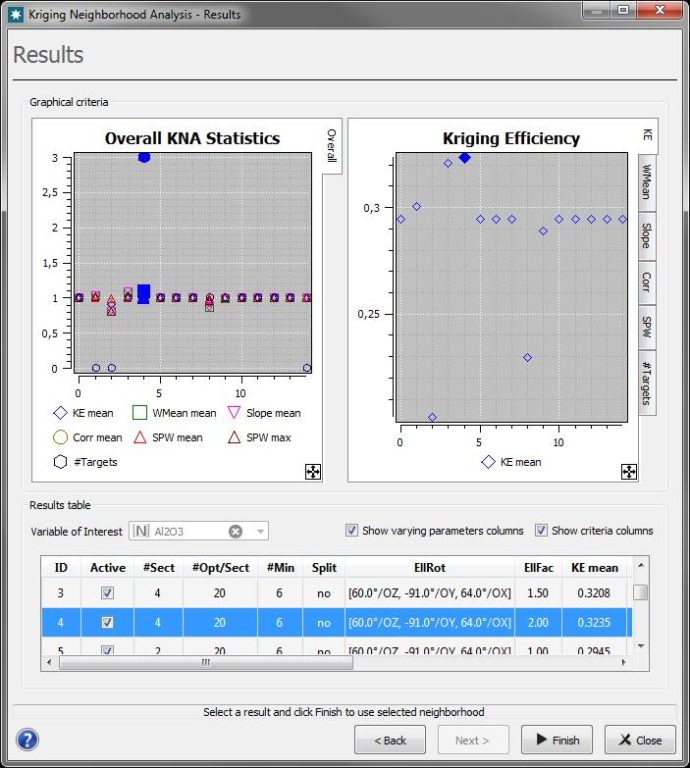
The dashed curve shows a spline that is smooth, but departs significantly from the expected values given by those means. The kriging interpolation, shown in red, runs along the means of the normally distributed credible intervals shown in gray. Squares indicate the location of the data. Example of one-dimensional data interpolation by kriging, with credible intervals.
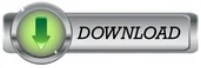